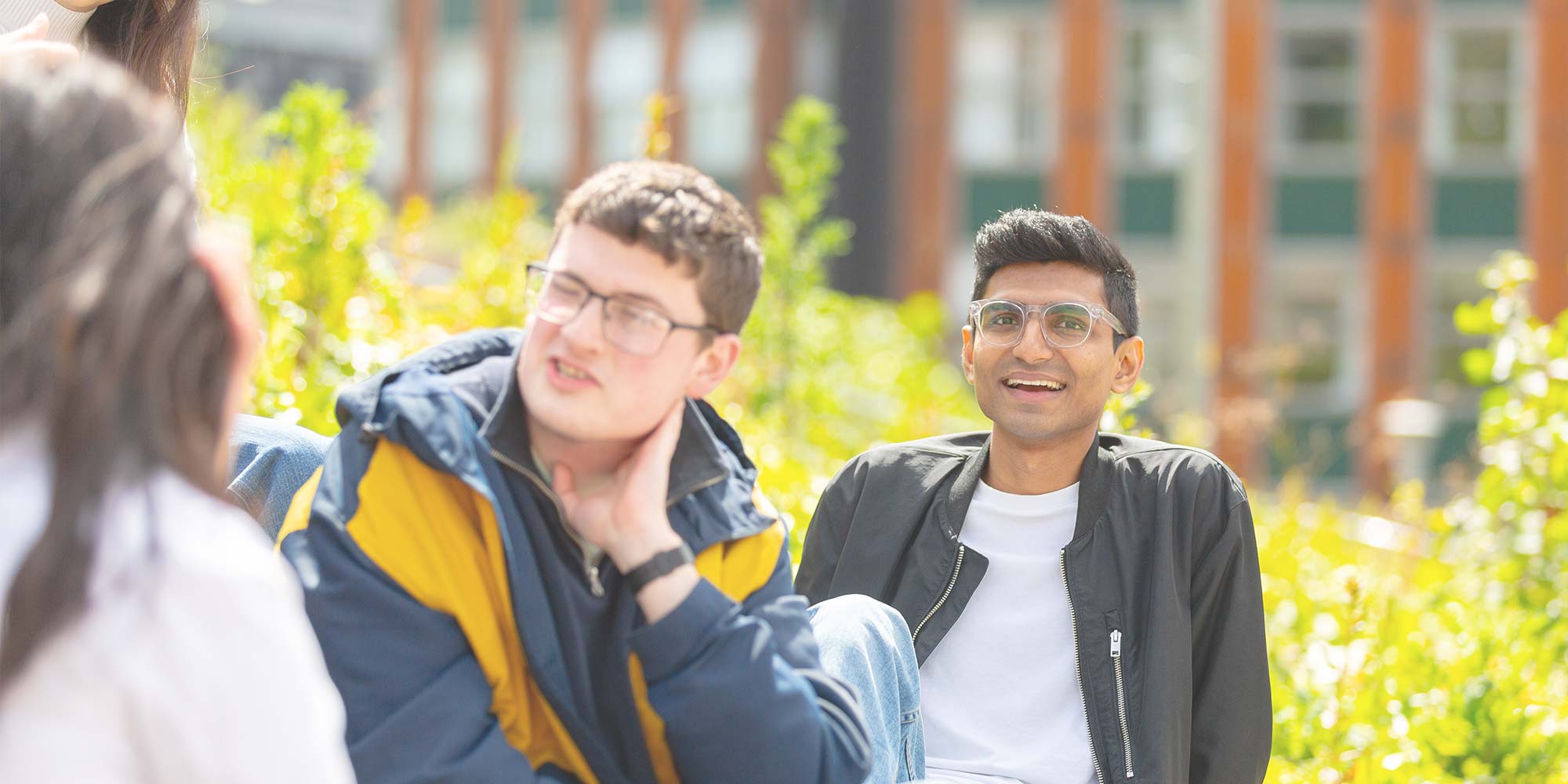
Strathclyde Game Changers - 2022 Summer Research Internships
ApplyKey facts
- Number of scholarships: 4
- Value: Fully Funded
- Opens: 3 February 2022
- Deadline: 16 February 2022
- Helps with: Living costs
- Duration: 8 to 12 weeks
Summary
The University of Strathclyde is constantly looking to improve its research output and working environments. A key aspect of this is ensuring we build teams made up of diverse groups of thinkers and problem solvers, collaborating in supportive and inclusive environments. We know that the best results are achieved when different voices and different points of view are all brought together. In order to foster the next generation of game changing researchers, we have therefore established a program of internships to demystify research and ensure the possibilities of academia are open to all.
Project details
Projects 1 and 2 are detailed below, and project 3 and 4 will be announced shortly. Please check the details on the How To Apply section for information on how to apply for these two projects, and how to express your interest for the subsequent projects.
Project 1: Characterising electrical fault manifestation of integrated systems for future aircraft
Background and motivation: Sustainable transport, including aviation, is recognized by the United Nations as key to sustainable development, enhancing economic growth and social equity, and directly supports UN Sustainable Development Goals 9 and 13. Disruptive technologies are needed to meet net-zero aviation targets, with advances in technology, operations and fuels combining to realise net-zero aviation. Lightweight structures (carbon fibre reinforced polymer (CFRP)) and electrification are two key enabling technologies to support these areas.
Integrated CFRP and electrical power systems (where the electrical power system and cables are in direct contact with, routed through or encased in CFRP) offer a route to significantly reducing the weight of the onboard electrical power system. This offers pathways to new design opportunities, by reducing the required power density of other electrical power system equipment, in turn enabling net-zero targets to be met.
Due to the safety critical nature of the aircraft application, it is essential that any degradation of the integrated system is detected as early as possible. Aircraft operate in a highly hazardous environment: extreme vibration, temperature, and low pressure. All of which can lead to degradation of on-board systems, and electrical fault scenarios where electrical current passes through CFRP. The low electrical conductivity of CFRP results in localised heating and structural damage of the CFRP, even at relatively low levels of power dissipation. This project will investigate the process of fault manifestation in these systems. It will develop experimental methodologies, and associated measurement and capture of data sets of the degradation process, when caused by vibration, which can be utilised in the longer term to train models for health monitoring and prognostics.
What will you do? The aim of this project is to develop experimental methodologies to capture the manifestation of electrical faults in integrated CFRP electrical power systems. This project will:
- From the published literature, map out the fault manifestation processes due to vibration, and review prognostic health monitoring data sets to understand experimental approaches to gather data sets for model training, including data capture whilst the system under test is subjected to vibration
- Carry out sets of experiments to gather data for analysis of the fault manifestation process
- Capture an exemplar data set for general use across the group with a view to publication, including pulling together documentation
- Provide an opportunity to gain experience of academic research, working in a collaborative team environment, contributing to both the Aero-electrical and Intelligent Systems Teams, and developing experimental hardware
Required Skills and experience: Candidates should have completed their 3rd or 4th (if going into 5th year, MEng) year of an undergraduate degree which includes Electrical Engineering (joint honours with other engineering disciplines is welcomed), have an interest in experimental work and metrology, and have independent initiative to help develop the project within a supportive team environment. Candidates should also be able to communicate results of their research to other members of the project team in informal discussions and more formal written reports and presentations. Skills in data capture methods, and previous experience of laboratory experimental work is desired, but not essential.
Project 2: Deep Learning based automated defect recognition for laser ultrasonic imaging
Background and motivation: Ultrasonics is a method to image the inner part of an optically opaque component and it has found a wide range of uses in applications ranging from medicine to aerospace. Laser ultrasonics is a technique where a pulsed laser is used to generate a high frequency sound (ultrasound) which propagates through the material and is detected by another laser. Laser ultrasound has the unique advantage of being a remote ultrasonic technique, making it suitable for extreme environments, such as the hot environment of a manufacturing process or even space.
Ultrasonic imaging based on laser ultrasonic data has been made possible, however, the technique is currently very slow to be of interest in practical applications. Applying automated, ultrasonic imaging analysis, on-the-fly, during the data acquisition stage, would be a game changer, allowing the technique to be applied to situations such as process monitoring during additive manufacturing (metal 3D printing), welding or in vivo medical scanning. One way to achieve automated imaging analysis is through machine learning. Deep Learning is a machine learning technique that uses neural networks to identify patterns in data. The research related to this project will help us answer the following question:
Can we use Deep Learning to train the system and identify "defects" in the acquired ultrasonic images, without human intervention?
If we achieved this, we would unlock the application potential of the technique for a wide range of industrial cases.
What will you do? The project will first review the existing Deep Learning algorithms and decide on suitability for data provided from laser ultrasonics. You will generate, synthetic ultrasonic data using the existing laser ultrasonic model of a commercial finite element package (Onscale). These synthetic data-sets will be used to compose ultrasonic images, using existing ultrasonic algorithms, implemented through MATLAB. Some of these images will have defects and some will be defect-free and they will be used as the "ground truth" to train the Deep Learning algorithm. Finally, the trained network will be used on experimental data to determine if they are defect free or not. You will be supported, through all these stages, working closely as a member of an active and collaborative research team.
Required skills and experience: We are seeking candidates with enthusiasm for research and data processing that will have just finished their 3rd or 4th year of study, by the end of the internship. An understanding of machine learning is essential. An understanding of ultrasonic signals and image processing is desirable but not essential. Prior knowledge in programming using MATLAB would be an added advantage. Candidates should enjoy working as part of a team. The internship is proposed for a duration of 8 weeks.
How to apply
Four funded places are currently available for summer 2022. Two of these projects are detailed under the Project Details section. Information on the remaining two will be available soon.
In order to apply for Projects 1 or 2, please submit an up-to-date CV and covering letter which details
- why you are excited by this project, and
- why you are keen to participate in a scheme designed to help improve the diversity and inclusiveness of research teams.
In order to request the details of Projects 3 and 4 (when available), please register your interest using the email address given below.
Application documents or registrations-of-interest should be submitted to edward.hart@strath.ac.uk as soon as possible.
The submission deadline for Projects 1 and 2 is Weds 16th February 2022, with interviews to be held on the 21st and 22nd February.
Contact us
Please contact Dr Edward Hart (edward.hart@strath.ac.uk) with any queries related to this internship opportunity.